Uncertainty, Behavior, and the Limits of Rationality

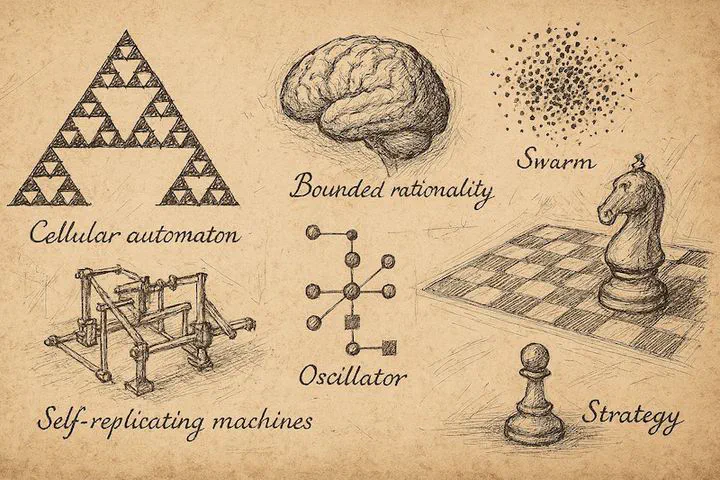
Better decisions often come from better data, and executives are right to rely on it. But in many real-world scenarios, decisions are made under uncertainty, in reaction to others… competitors, customers, regulators, teams. This is where ๐ฏ๐ฒ๐ต๐ฎ๐๐ถ๐ผ๐ฟ๐ฎ๐น ๐๐ฐ๐ถ๐ฒ๐ป๐ฐ๐ฒ and the idea of ๐ฏ๐ผ๐๐ป๐ฑ๐ฒ๐ฑ ๐ฟ๐ฎ๐๐ถ๐ผ๐ป๐ฎ๐น๐ถ๐๐ come in. People ๐ช๐ฏ๐ต๐ฆ๐ฏ๐ฅ to make good decisions, but whatโs โgoodโ depends on what they know, how much time they have, and what they expect others might do.
In last nightโs MTAC** session, we presented similar situations and asked graduate students to model and reconstruct the resulting phenomena from agents making decisions.
We began with thought exercises. No code at first, just their sense of how the systems might behave. When they shared their models, we asked: ๐๐ข๐ฏ ๐ต๐ฉ๐ข๐ต ๐ญ๐ฐ๐จ๐ช๐ค ๐ฃ๐ฆ ๐ค๐ฐ๐ฅ๐ช๐ง๐ช๐ฆ๐ฅ? ๐๐ข๐ฏ ๐ช๐ต ๐ฃ๐ฆ ๐ฆ๐น๐ฑ๐ณ๐ฆ๐ด๐ด๐ฆ๐ฅ ๐ช๐ฏ ๐ต๐ฉ๐ฆ ๐ญ๐ข๐ฏ๐จ๐ถ๐ข๐จ๐ฆ ๐ฐ๐ง ๐ด๐บ๐ด๐ต๐ฆ๐ฎ๐ดโ๐ต๐ฉ๐ฆ ๐ญ๐ข๐ฏ๐จ๐ถ๐ข๐จ๐ฆ ๐ฐ๐ง ๐ฎ๐ข๐ต๐ฉ๐ฆ๐ฎ๐ข๐ต๐ช๐ค๐ด? That shift from intuition to formalism is part of the real work of data scientists.
They all looked at the same scenarios. But every model came out different. That says something. Computational modeling is technical, but itโs also about framing. What matters? What can you ignore? What do you assume people will actually do?
Later, we explored ๐ช๐ผ๐น๐ณ๐ฟ๐ฎ๐บโ๐ ๐ฐ๐ฒ๐น๐น๐๐น๐ฎ๐ฟ ๐ฎ๐๐๐ผ๐บ๐ฎ๐๐ฎ and ๐๐ผ๐ป๐๐ฎ๐โ๐ ๐๐ฎ๐บ๐ฒ ๐ผ๐ณ ๐๐ถ๐ณ๐ฒ. A few simple rules produced behaviors that were complex and often counterintuitive.
These exercises remind us that many systems, from markets to organizations and even teams, emerge from local decisions shaped by interactions and reactions to others. We operate within constraints, using partial views of the whole. Modeling gives us a way to surface those interactions and ask better questions about how structure forms… not by design but through behaviors.
** ๐๐๐๐๐ ๐โ๐๐๐๐๐๐, ๐ด๐๐๐๐๐๐กโ๐๐ , ๐๐๐ ๐ถ๐๐๐๐ข๐ก๐๐ก๐๐๐๐