The Monty Hall Problem in Digital Transformation: Overcoming Cognitive Biases

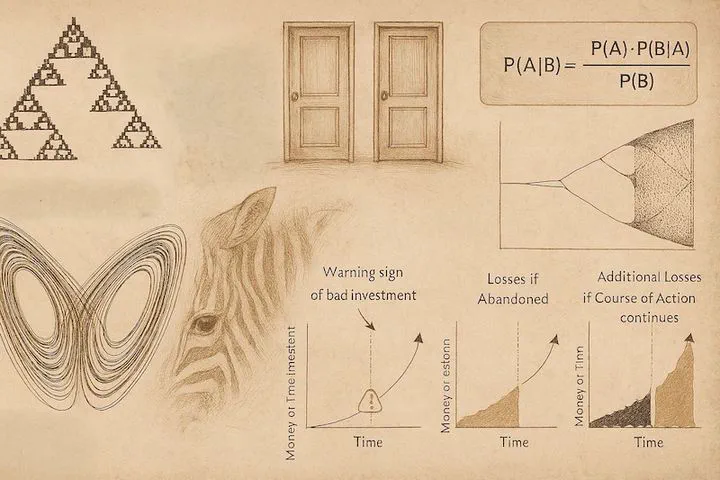
We like to believe weโre rational decision-makers, especially when leading digital initiatives. This has been my consistent observation. Yet two subtle cognitive habits often shape our choices more than data ever could. (๐๐ง ๐ค๐ฐ๐ถ๐ณ๐ด๐ฆ, ๐ณ๐ฆ๐ข๐ญ-๐ธ๐ฐ๐ณ๐ญ๐ฅ ๐ฅ๐ฆ๐ค๐ช๐ด๐ช๐ฐ๐ฏ-๐ฎ๐ข๐ฌ๐ช๐ฏ๐จ ๐ช๐ด ๐ง๐ข๐ณ ๐ฎ๐ฐ๐ณ๐ฆ ๐ค๐ฐ๐ฎ๐ฑ๐ญ๐ฆ๐น ๐ต๐ฉ๐ข๐ฏ ๐ข๐ฏ๐บ ๐ง๐ณ๐ข๐ฎ๐ฆ๐ธ๐ฐ๐ณ๐ฌ ๐ค๐ข๐ฏ ๐ง๐ถ๐ญ๐ญ๐บ ๐ค๐ข๐ฑ๐ต๐ถ๐ณ๐ฆ, ๐ฃ๐ถ๐ต ๐ต๐ฉ๐ฆ๐ด๐ฆ ๐ฑ๐ข๐ต๐ต๐ฆ๐ณ๐ฏ๐ด ๐ข๐ณ๐ฆ ๐ด๐ต๐ช๐ญ๐ญ ๐ธ๐ฐ๐ณ๐ต๐ฉ ๐ฆ๐น๐ข๐ฎ๐ช๐ฏ๐ช๐ฏ๐จ.)
Take the Monty Hall problem. Three doors. Behind one is a prize. You pick one. The host opens another and shows itโs empty. Youโre asked: stick or switch?
Most people stick. But Bayesian reasoning tells us to switch.
Why donโt we?
Now think of the sunk cost fallacy. Youโve spent a year on a project. Time, money, energy. Itโs not working. But instead of letting go, you keep going. Because of everything youโve already invested.
Why?
While these examples simplify complex decision processes, they highlight patterns that emerge even in sophisticated business contexts. Both situations feel different, but the thinking is the same. We commit early. We ignore new information. We assume our first decision is better than it is.
Iโve been working with a PhD in DS student on nonlinear dynamics. We are exploring how simple rules give rise to complex behaviors. Weโre combining DL techniques and the classic cellular automata to build something more meta: a model that predicts which kinds of frameworks are likely to produce the patterns we see. In a sense, weโre modeling the model. This project has become a perfect laboratory for observing our own cognitive biases in action.
The academic project seems ambitious. And after a year, weโre still stuck. ๐ The sub-models work. But the overall picture is still fuzzy. Still, itโs tempting to keep pushing forwardโฆ to avoid stepping back, questioning our assumptions, or shifting our frame.
But building models is only part of the work. The harder part is recognizing when the model no longer fits. When the structure we started with isnโt capturing what matters. When itโs time to ask: should we shift? Should we reframe?
Now back to digital transformation, sunk costs show up as legacy systems, outdated processes, or half-built models weโre afraid to stop using. Can we switch doors when the data tells us to?
Sure, the original idea may have opened the door. But progress sometimes comes from walking away and trying another. That said, there are certainly cases where persistence through difficulties leads to breakthrough. And distinguishing between productive perseverance and the sunk cost fallacy is the real art! Just like tuning the hyper-parameters of our deep learning models ๐